6. Reading Data into a DataFrame
Reading Data into a DataFrame
Pandas provides functions for reading data from various formats, for example:
- read_csv
- read_json
- read_table
- read_clipboard
- read_excel
- read_html
- read_sql
These functions offer many optional parameters that can be used to:
- Define DataFrame indexes (row and column headers)
- Specify transformations for the data
- Parse dates, for example, based on the values in different columns
- Determine how missing values or dirty data are handled
read_csv()
The read_csv()
function of the Pandas library reads data from a text file where records are separated by delimiters. The default delimiter is a comma, but it can be changed with the delimiter parameter.
The read_csv()
function has about 50 possible parameters, but the only mandatory one is the file name, path, or URL.
The example file contains the lines:
The file can be imported from a local hard drive or directly from a website using its address.
NOTE! All datasets can be found at https://student.labranet.jamk.fi/~rmika/Datasets/
import pandas as pd
# Reading from a file on a local hard drive
# The file can be downloaded to your own computer from: https://student.labranet.jamk.fi/~rmika/Datasets/data-w3.csv
# Move the file to the same directory as the Notebook or path it to the correct location,
# e.g., pd.read_csv('../Datasets/data-w3.csv')
df = pd.read_csv('data-w3.csv')
df
<style>
.dataframe thead th {
text-align: right;
}
</style>
<table border="1" class="dataframe">
<thead>
<tr style="text-align: right;">
<th></th>
<th>duration</th>
<th>pulse</th>
<th>max_pulse</th>
<th>calories</th>
</tr>
</thead>
<tbody>
<tr>
<th>0</th>
<td>60</td>
<td>110</td>
<td>130</td>
<td>409.1</td>
</tr>
<tr>
<th>1</th>
<td>60</td>
<td>117</td>
<td>145</td>
<td>479.0</td>
</tr>
<tr>
<th>2</th>
<td>60</td>
<td>103</td>
<td>135</td>
<td>340.0</td>
</tr>
<tr>
<th>3</th>
<td>45</td>
<td>109</td>
<td>175</td>
<td>282.4</td>
</tr>
<tr>
<th>4</th>
<td>45</td>
<td>117</td>
<td>148</td>
<td>406.0</td>
</tr>
<tr>
<th>...</th>
<td>...</td>
<td>...</td>
<td>...</td>
<td>...</td>
</tr>
<tr>
<th>164</th>
<td>60</td>
<td>105</td>
<td>140</td>
<td>290.8</td>
</tr>
<tr>
<th>165</th>
<td>60</td>
<td>110</td>
<td>145</td>
<td>300.0</td>
</tr>
<tr>
<th>166</th>
<td>60</td>
<td>115</td>
<td>145</td>
<td>310.2</td>
</tr>
<tr>
<th>167</th>
<td>75</td>
<td>120</td>
<td>150</td>
<td>320.4</td>
</tr>
<tr>
<th>168</th>
<td>75</td>
<td>125</td>
<td>150</td>
<td>330.4</td>
</tr>
</tbody>
</table>
<p>169 rows × 4 columns</p>
</div>
The `usecols` parameter can be used to select only the desired columns.
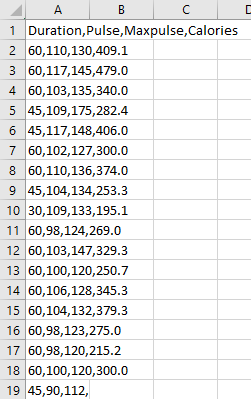
```python
df = pd.read_csv('Datasets/data-w3.csv', sep=',', decimal='.',
usecols=['Duration', 'Pulse'])
df
ip | date | timezone | request | status | size | referer | user_agent | |
---|---|---|---|---|---|---|---|---|
0 | 109.169.248.247 | [12/Dec/2015:18:25:11 | +0100] | GET /administrator/ HTTP/1.1 | 200 | 4263 | - | Mozilla/5.0 (Windows NT 6.0; rv:34.0) Gecko/20... |
1 | 109.169.248.247 | [12/Dec/2015:18:25:11 | +0100] | POST /administrator/index.php HTTP/1.1 | 200 | 4494 | http://almhuette-raith.at/administrator/ | Mozilla/5.0 (Windows NT 6.0; rv:34.0) Gecko/20... |
2 | 46.72.177.4 | [12/Dec/2015:18:31:08 | +0100] | GET /administrator/ HTTP/1.1 | 200 | 4263 | - | Mozilla/5.0 (Windows NT 6.0; rv:34.0) Gecko/20... |
3 | 46.72.177.4 | [12/Dec/2015:18:31:08 | +0100] | POST /administrator/index.php HTTP/1.1 | 200 | 4494 | http://almhuette-raith.at/administrator/ | Mozilla/5.0 (Windows NT 6.0; rv:34.0) Gecko/20... |
4 | 83.167.113.100 | [12/Dec/2015:18:31:25 | +0100] | GET /administrator/ HTTP/1.1 | 200 | 4263 | - | Mozilla/5.0 (Windows NT 6.0; rv:34.0) Gecko/20... |
[
{
"firstName":"Helen",
"lastName":"Maroulis",
"age":32,
"active":true
}
]
[
{
"firstName":"Kaori",
"lastName":"Icho",
"age":38,
"active":false
},
{
"firstName":"Helen",
"lastName":"Maroulis",
"age":32,
"active":true
}
] ```
JSON was created as an alternative to XML, which was once the dominant form of data exchange. XML files are difficult for humans to read and leave much to be desired in terms of coding agility. JSON was the answer to these problems.
```python
# Reading a JSON object
df = pd.read_json('Datasets/participant-simple.json')
df
firstname | lastname | age | active | |
---|---|---|---|---|
0 | Kaori | Icho | 38 | False |
1 | Helen | Maroulis | 32 | True |
{
"firstname":"Helen",
"lastname":"Maroulis",
"age":32,
"achievements":[
{
"olympics":"Rio De Janeiro",
"country":"Brazil",
"placement":"gold",
"weight class":"57 kg",
"year":2016
}
],
"active":true
}```
```python
# Reading a JSON object
df = pd.read_json('Datasets/participant-deep.json')
df
firstname | lastname | age | achievements | active | |
---|---|---|---|---|---|
0 | Helen | Maroulis | 32 | {'olympics': 'Rio De Janeiro', 'country': 'Br... | True |
df.info()
import json
with open('Datasets/participant-deep.json','r') as f:
data = json.loads(f.read())
# Flatten data
df_list = pd.json_normalize(data, record_path =['achievements'])
df_list
olympics | country | placement | weight class | year | |
---|---|---|---|---|---|
0 | Rio De Janeiro | Brazil | gold | 57 kg | 2016 |
import json
with open('Datasets/participant-deep.json','r') as f:
data = json.loads(f.read())
# Flatten data
df_list = pd.json_normalize(
data,
record_path =['achievements'],
meta=['firstname', 'lastname']
)
df_list
olympics | country | placement | weight class | year | firstname | lastname | |
---|---|---|---|---|---|---|---|
0 | Rio De Janeiro | Brazil | gold | 57 kg | 2016 | Helen | Maroulis |
import requests
url= "https://tie.digitraffic.fi/api/v1/data/tms-data/23001"
req = requests.get(url=url)
req.status_code
cat_data = req.json()
cat_data
{
"dataUpdatedTime": "2022-11-14T09:36:35Z",
"tmsStations": [
{
"id": 23001,
"tmsNumber": 1,
"measuredTime": "2022-11-14T09:36:30Z",
"sensorValues": [
{
"id": 5054,
"roadStationId": 23001,
"name": "PASSES_60MIN_FIXED_DIRECTION1",
"oldName": "passes_60min_fixed_direction1",
"shortName": "pcs/h1",
"sensorValue": 417.0,
"sensorUnit": "pcs/h",
"timeWindowStart": "2022-11-14T08:00:00Z",
"timeWindowEnd": "2022-11-14T09:00:00Z",
"measuredTime": "2022-11-14T09:36:30Z"
},
{
"id": 5055,
"roadStationId": 23001,
"name": "PASSES_60MIN_FIXED_DIRECTION2",
"oldName": "passes_60min_fixed_direction2",
"shortName": "pcs/h2",
"sensorValue": 304.0,
"sensorUnit": "pcs/h",
"timeWindowStart": "2022-11-14T08:00:00Z",
"timeWindowEnd": "2022-11-14T09:00:00Z",
"measuredTime": "2022-11-14T09:36:30Z"
},
{
"id": 5056,
"roadStationId": 23001,
"name": "AVERAGE_SPEED_60MIN_FIXED_DIRECTION1",
"oldName": "average_speed_60min_fixed_direction1",
"shortName": "km/h1",
"sensorValue": 107.0,
"sensorUnit": "km/h",
"timeWindowStart": "2022-11-14T08:00:00Z",
"timeWindowEnd": "2022-11-14T09:00:00Z",
"measuredTime": "2022-11-14T09:36:30Z"
},
{
"id": 5057,
"roadStationId": 23001,
"name": "AVERAGE_SPEED_60MIN_FIXED_DIRECTION2",
"oldName": "average_speed_60min_fixed_direction2",
"shortName": "km/h2",
"sensorValue": 108.0,
"sensorUnit": "km/h",
"timeWindowStart": "2022-11-14T08:00:00Z",
"timeWindowEnd": "2022-11-14T09:00:00Z",
"measuredTime": "2022-11-14T09:36:30Z"
},
{
"id": 5058,
"roadStationId": 23001,
"name": "AVERAGE_SPEED_5MIN_FIXED_DIRECTION1_FREEFLOW1",
"oldName": "average_speed_5min_fixed_direction1_FREEFLOW1",
"shortName": "FCond1",
"sensorValue": 116.0,
"sensorUnit": "***",
"timeWindowStart": "2022-11-14T09:30:00Z",
"timeWindowEnd": "2022-11-14T09:35:00Z",
"measuredTime": "2022-11-14T09:36:30Z"
},
{
"id": 5061,
"roadStationId": 23001,
"name": "AVERAGE_SPEED_5MIN_FIXED_DIRECTION2_FREEFLOW2",
"oldName": "average_speed_5min_fixed_direction2_FREEFLOW2",
"shortName": "FCond2",
"sensorValue": 105.0,
"sensorUnit": "***",
"timeWindowStart": "2022-11-14T09:30:00Z",
"timeWindowEnd": "2022-11-14T09:35:00Z",
"measuredTime": "2022-11-14T09:36:30Z"
},
{
"id": 5064,
"roadStationId": 23001,
"name": "PASSES_5MIN_FIXED_DIRECTION1_MS1",
"oldName": "passes_5min_fixed_direction1_MS1",
"shortName": "MCond1",
"sensorValue": 16.0,
"sensorUnit": "***",
"timeWindowStart": "2022-11-14T09:30:00Z",
"timeWindowEnd": "2022-11-14T09:35:00Z",
"measuredTime": "2022-11-14T09:36:30Z"
},
{
"id": 5067,
"roadStationId": 23001,
"name": "PASSES_60MIN_FIXED_DIRECTION1_MS1",
"oldName": "passes_60min_fixed_direction1_MS1",
"shortName": "MCond1",
"sensorValue": 15.0,
"sensorUnit": "***",
"timeWindowStart": "2022-11-14T08:00:00Z",
"timeWindowEnd": "2022-11-14T09:00:00Z",
"measuredTime": "2022-11-14T09:36:30Z"
},
{
"id": 5068,
"roadStationId": 23001,
"name": "PASSES_5MIN_FIXED_DIRECTION2_MS2",
"oldName": "passes_5min_fixed_direction2_MS2",
"shortName": "MCond2",
"sensorValue": 10.0,
"sensorUnit": "***",
"timeWindowStart": "2022-11-14T09:30:00Z",
"timeWindowEnd": "2022-11-14T09:35:00Z",
"measuredTime": "2022-11-14T09:36:30Z"
},
{
"id": 5071,
"roadStationId": 23001,
"name": "PASSES_60MIN_FIXED_DIRECTION2_MS2",
"oldName": "passes_60min_fixed_direction2_MS2",
"shortName": "MCond2",
"sensorValue": 11.0,
"sensorUnit": "***",
"timeWindowStart": "2022-11-14T08:00:00Z",
"timeWindowEnd": "2022-11-14T09:00:00Z",
"measuredTime": "2022-11-14T09:36:30Z"
},
{
"id": 5116,
"roadStationId": 23001,
"name": "PASSES_5MIN_ROLLING_DIRECTION1",
"oldName": "trafficVolume1",
"shortName": "pcs/h1",
"sensorValue": 420.0,
"sensorUnit": "pcs/h",
"measuredTime": "2022-11-14T09:36:30Z"
},
{
"id": 5119,
"roadStationId": 23001,
"name": "PASSES_5MIN_ROLLING_DIRECTION2",
"oldName": "trafficVolume2",
"shortName": "pcs/h2",
"sensorValue": 276.0,
"sensorUnit": "pcs/h",
"measuredTime": "2022-11-14T09:36:30Z"
},
{
"id": 5122,
"roadStationId": 23001,
"name": "AVERAGE_SPEED_5MIN_ROLLING_DIRECTION1",
"oldName": "averageSpeed1",
"shortName": "km/h1",
"sensorValue": 110.0,
"sensorUnit": "km/h",
"measuredTime": "2022-11-14T09:36:30Z"
},
{
"id": 5125,
"roadStationId": 23001,
"name": "AVERAGE_SPEED_5MIN_ROLLING_DIRECTION2",
"oldName": "averageSpeed2",
"shortName": "km/h2",
"sensorValue": 109.0,
"sensorUnit": "km/h",
"measuredTime": "2022-11-14T09:36:30Z"
},
{
"id": 5158,
"roadStationId": 23001,
"name": "AVERAGE_SPEED_5MIN_ROLLING_DIRECTION1_FREEFLOW1",
"oldName": "average_speed_5min_rolling_direction1_FREEFLOW1",
"shortName": "FCond1",
"sensorValue": 116.0,
"sensorUnit": "***",
"measuredTime": "2022-11-14T09:36:30Z"
},
{
"id": 5161,
"roadStationId": 23001,
"name": "AVERAGE_SPEED_5MIN_ROLLING_DIRECTION2_FREEFLOW2",
"oldName": "average_speed_5min_rolling_direction2_FREEFLOW2",
"shortName": "FCond2",
"sensorValue": 103.0,
"sensorUnit": "***",
"measuredTime": "2022-11-14T09:36:30Z"
},
{
"id": 5164,
"roadStationId": 23001,
"name": "PASSES_5MIN_ROLLING_DIRECTION1_MS1",
"oldName": "passes_5min_rolling_direction1_MS1",
"shortName": "MCond1",
"sensorValue": 15.0,
"sensorUnit": "***",
"measuredTime": "2022-11-14T09:36:30Z"
},
{
"id": 5168,
"roadStationId": 23001,
"name": "PASSES_5MIN_ROLLING_DIRECTION2_MS2",
"oldName": "passes_5min_rolling_direction2_MS2",
"shortName": "MCond2",
"sensorValue": 10.0,
"sensorUnit": "***",
"measuredTime": "2022-11-14T09:36:30Z"
}
]
}
]
}
df = pd.read_csv('Datasets/data-w3-noHeader.csv', names=['duration', 'heart_rate', 'max_heart_rate', 'calories'])
df